Aram-Alexandre Pooladian
Center for Data Science, New York University
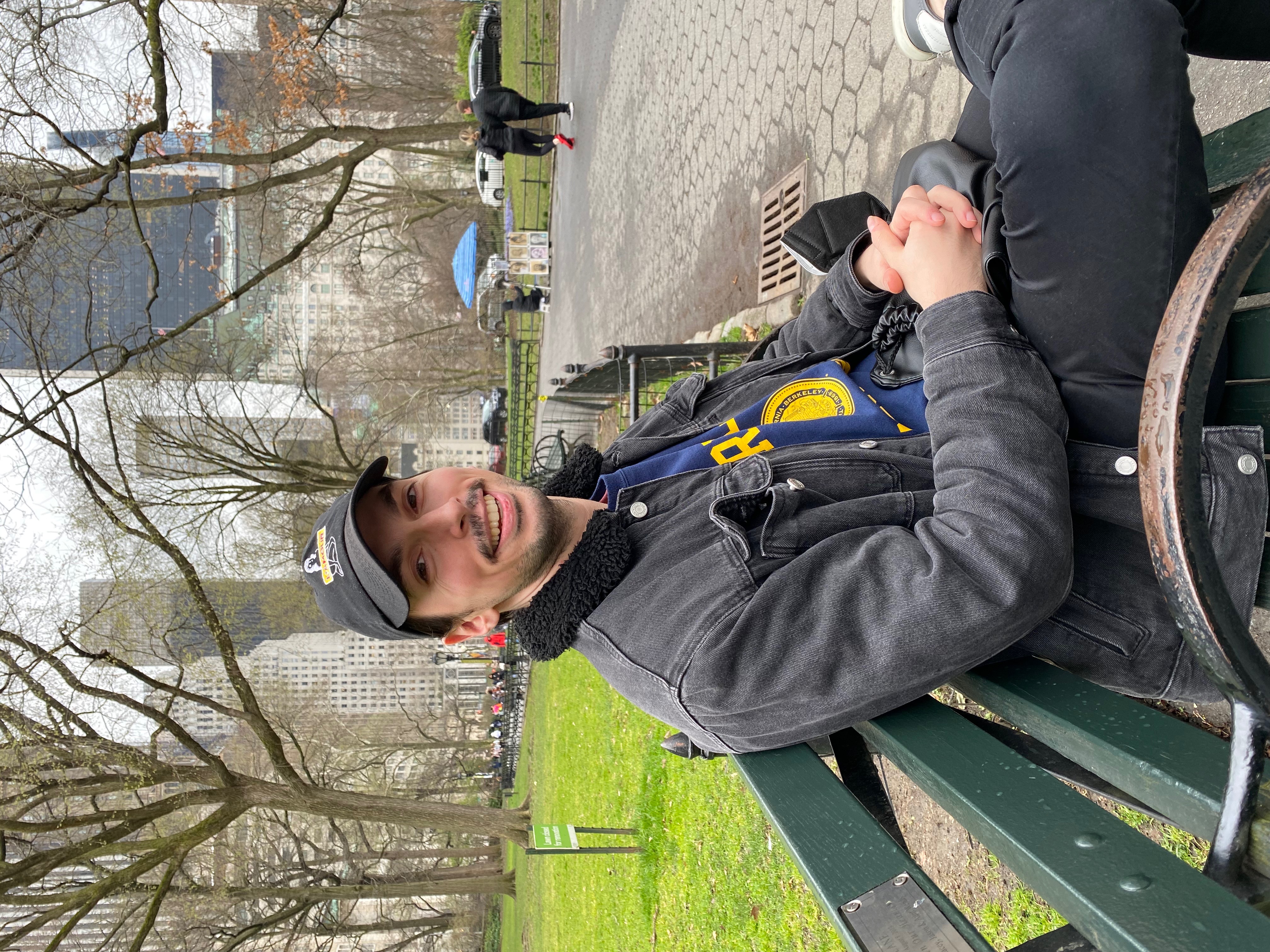
I am a final-year PhD student at the Center for Data Science at New York University, where I am fortunate to be advised by Jonathan Niles-Weed. In Fall 2025, I’ll be joining Yale University as an FDS postdoc. My research has been supported by NSF, NSERC, Google, and Meta AI Research.
I develop and analyze principled algorithms for large-scale probabilistic inference. Specifically, my research aims to bring mathematical and statistical rigor to empirically successful methodologies for contemporary statistical challenges, such as variational inference and diffusion models for generative modeling, among others. To achieve this, I have leveraged the rich theory of optimal transport—a discipline rooted in probability theory, partial differential equations, and geometry—as a foundational framework to understand the mathematics of data science.
Email: aram-alexandre[dot]pooladian[at]nyu[dot]edu
news
Feb 14, 2025 | Matthew Zhang copied my website template! |